Researchers at the University of Alberta offer insights into mental and physical health trajectories
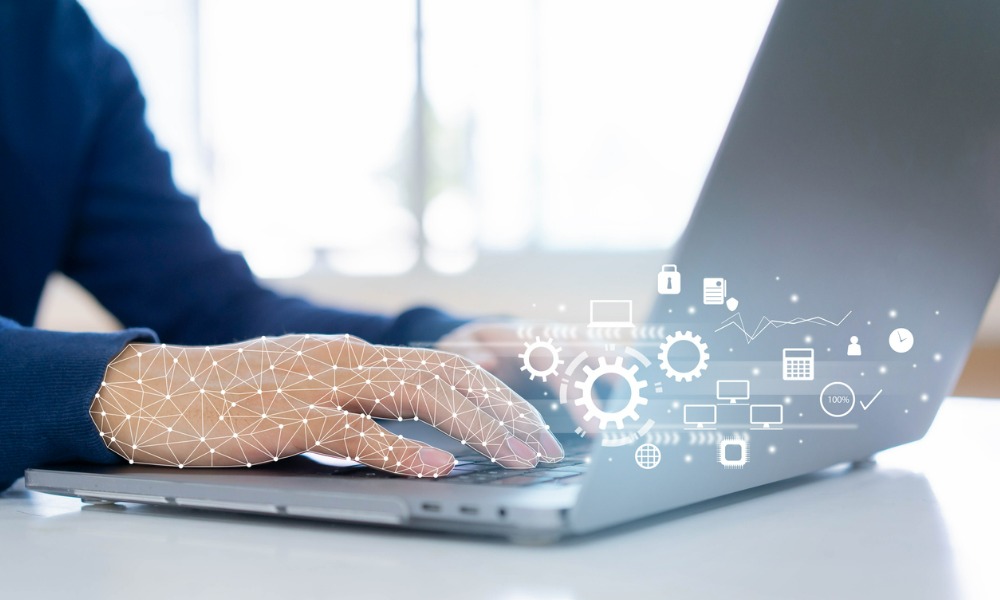
Researchers from the University of Alberta have utilized health-related, lifestyle, socio-economic, and other data to create machine learning programs that predict the future mental and physical health of aging Canadians.
Principal investigator Bo Cao, associate professor of psychiatry, adjunct professor of computing science, and Canada Research Chair in Computational Psychiatry, highlights the potential of machine learning in assisting health-care teams. “Machine learning is a powerful and useful computational method to utilize rich de-identified data,” says Cao.
“If we want to push for future individualized patient prediction for certain health outcomes, we need to leverage machine learning techniques,” he says.
Cao's team applied machine learning in two recently published studies using data from the Canadian Longitudinal Study on Aging (CLSA) involving over 30,000 Canadians aged 45 to 85. “Our goal is to contribute to the health of Albertans and Canadians. We hope to develop a healthy aging trajectory for every one of us,” Cao adds.
Health and aging
In the first study, the team developed a biological age index by applying machine learning models to blood test data from the CLSA. The study revealed that individuals may exhibit a physiological age distinct from their chronological age, suggesting a potential variance in the aging process. The researchers identified a “BioAge gap” and explored its associations with lifestyle, environmental factors, and health conditions.
The team reported strong associations between a positive BioAge gap (meaning older than chronological age) and chronic illness, certain dietary habits, smoking, and passive exposure to smoke. Additionally, the researchers identified modifiable factors, such as the consumption of fruits, legumes, and vegetables, which were linked to a negative BioAge gap (meaning younger than chronological age).
“Understanding these associations and identifying risk factors for differential aging could guide effective public health recommendations to promote healthy longevity,” the paper states.
Cao anticipates that understanding the most crucial factors or combinations of factors affecting the biological aging process will be the next focus of their research efforts.
Mental health
In the second study, the team developed a program predicting depression onset within three years, with about 70 percent accuracy. The model identified key predictors, including “existing subthreshold depression symptoms, emotional instability, low levels of life satisfaction, perceived health and social support, and nutrition risk as the most important predictors for depression onset,” the paper states.
“It’s interesting that even using only personality measures, perceived health or mental health, nutrition and other factors not directly relevant to depressive symptoms and stress, depression can still be predicted,” Cao says.
Cao acknowledges that both the mental health machine learning model and the BioAge model are not yet refined for real-world implementation. “We are trying to establish a conversation that includes different groups — clinicians, patients, and people with lived experience — to demonstrate this kind of model can benefit the general public.”